Credit Risk Modeling in Python
Learn how to prepare credit application data, apply machine learning and business rules to reduce risk and ensure profitability.
Start Course for Free4 hours15 videos57 exercises20,499 learnersStatement of Accomplishment
Create Your Free Account
or
By continuing, you accept our Terms of Use, our Privacy Policy and that your data is stored in the USA.Training 2 or more people?
Try DataCamp for BusinessLoved by learners at thousands of companies
Course Description
If you've ever applied for a credit card or loan, you know that financial firms process your information before making a decision. This is because giving you a loan can have a serious financial impact on their business. But how do they make a decision? In this course, you will learn how to prepare credit application data. After that, you will apply machine learning and business rules to reduce risk and ensure profitability. You will use two data sets that emulate real credit applications while focusing on business value. Join me and learn the expected value of credit risk modeling!
For Business
Training 2 or more people?
Get your team access to the full DataCamp library, with centralized reporting, assignments, projects and moreIn the following Tracks
Applied Finance in Python
Go To Track- 1
Exploring and Preparing Loan Data
FreeIn this first chapter, we will discuss the concept of credit risk and define how it is calculated. Using cross tables and plots, we will explore a real-world data set. Before applying machine learning, we will process this data by finding and resolving problems.
Understanding credit risk50 xpExplore the credit data100 xpCrosstab and pivot tables100 xpOutliers in credit data50 xpFinding outliers with cross tables100 xpVisualizing credit outliers100 xpRisk with missing data in loan data50 xpReplacing missing credit data100 xpRemoving missing data100 xpMissing data intuition50 xp - 2
Logistic Regression for Defaults
With the loan data fully prepared, we will discuss the logistic regression model which is a standard in risk modeling. We will understand the components of this model as well as how to score its performance. Once we've created predictions, we can explore the financial impact of utilizing this model.
Logistic regression for probability of default50 xpLogistic regression basics100 xpMultivariate logistic regression100 xpCreating training and test sets100 xpPredicting the probability of default50 xpChanging coefficients100 xpOne-hot encoding credit data100 xpPredicting probability of default100 xpCredit model performance50 xpDefault classification reporting100 xpSelecting report metrics100 xpVisually scoring credit models100 xpModel discrimination and impact50 xpThresholds and confusion matrices100 xpHow thresholds affect performance100 xpThreshold selection100 xp - 3
Gradient Boosted Trees Using XGBoost
Decision trees are another standard credit risk model. We will go beyond decision trees by using the trendy XGBoost package in Python to create gradient boosted trees. After developing sophisticated models, we will stress test their performance and discuss column selection in unbalanced data.
Gradient boosted trees with XGBoost50 xpTrees for defaults100 xpGradient boosted portfolio performance100 xpAssessing gradient boosted trees100 xpColumn selection for credit risk50 xpColumn importance and default prediction100 xpVisualizing column importance100 xpColumn selection and model performance100 xpCross validation for credit models50 xpCross validating credit models100 xpLimits to cross-validation testing100 xpCross-validation scoring100 xpClass imbalance in loan data50 xpUndersampling training data100 xpUndersampled tree performance100 xpUndersampling intuition50 xp - 4
Model Evaluation and Implementation
After developing and testing two powerful machine learning models, we use key performance metrics to compare them. Using advanced model selection techniques specifically for financial modeling, we will select one model. With that model, we will: develop a business strategy, estimate portfolio value, and minimize expected loss.
Model evaluation and implementation50 xpComparing model reports100 xpComparing with ROCs100 xpCalibration curves100 xpCredit acceptance rates50 xpAcceptance rates100 xpVisualizing quantiles of acceptance100 xpBad rates100 xpAcceptance rate impact100 xpCredit strategy and minimum expected loss50 xpMaking the strategy table100 xpVisualizing the strategy100 xpEstimated value profiling100 xpTotal expected loss100 xpCourse wrap up50 xp
For Business
Training 2 or more people?
Get your team access to the full DataCamp library, with centralized reporting, assignments, projects and moreIn the following Tracks
Applied Finance in Python
Go To Trackdatasets
Raw credit dataClean credit data (outliers and missing data removed)Credit data (ready for modeling)collaborators
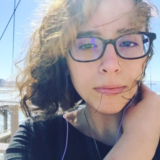
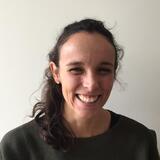
prerequisites
Intermediate Python for FinanceMichael Crabtree
See MoreData Scientist
What do other learners have to say?
Join over 15 million learners and start Credit Risk Modeling in Python today!
Create Your Free Account
or
By continuing, you accept our Terms of Use, our Privacy Policy and that your data is stored in the USA.