[Radar Recap] From Data Governance to Data Discoverability: Building Trust in Data Within Your Organization with Esther Munyi, Amy Grace, Stefaan Verhulst and Malarvizhi Veerappan
Esther oversees the data strategy, governance, and architecture at Sasfin. She is focused on developing and implementing cutting-edge data solutions, and establishing data as a core asset and enabler for Sasfin. Esther was recognized as the CDO of the Year 2023 at the FINNOVEX South Africa Awards, and as one of the Global Data Power Women in 2023.
Over her 35-year career with Raytheon Technologies, Amy’s passion has been applying new analysis techniques to deliver products with a measurable impact to customer satisfaction. Amy spent her first 28 years at Pratt & Whitney as a Systems Engineer before transitioning to Collins Aerospace in 2016 to lead development of aircraft predictive maintenance analytics for the A320 and 787 Dreamliner. Amy was inducted into the Collins Aerospace Fellows Program as Fellow, Applied Data Science. Amy returned to P&W in 2020 to lead development of cutting-edge digital solutions to support safe and affordable operation of Pratt & Whitney military engines. Amy received her BS degree in Aerospace Engineering from Syracuse University.
Stefaan founded The GovLab, a global action research center at New York University. His work focuses on how to transform the way society makes decisions leveraging data and new technologies, supervising a team of 20 researchers, and teaching executive courses on data stewardship and data governance. He is also a Research Professor at NYU Center for Urban Sciences + Progress, Co-Founder and the Principal Scientific Advisor at The Data Tank, and a Senior Advisor at Markle Foundation.
Malarvizhi (“Malar”) Veerappan is a Program Manager and Senior Data Scientist at the World Bank. Her expertise lies at the convergence of large-scale data governance, management, analytics, and technology implementations. Currently, her focus is on guiding countries' mainstream digital solutions and data in health system transformation engagements. As a coauthor of the "Digital-in-Health: Unlocking Value for Everyone" report and the report manager for the 2021 World Development Report – "Data for Better Lives," she brings a wealth of experience gained from collaborating with countries across Africa, Asia, Latin America, and Europe, as well as various organizations. Her interest lies in finding innovative solutions that leverage data and technology to address development challenges. She has led large scale data initiatives including the modernization of the World Bank’s data management and dissemination architecture encompassing policies, systems and processes. She was part of the task team that launched The World Bank's Open Data Initiative and was instrumental in creating the World Bank’s first Data Council, the data governance body set up to prioritize the institution's key data priorities. As part of this work, she created the Bank’s data management architecture and established the Development Data Hub, the Bank’s first integrated data hub, that streamlines data sharing and breaks down data silos by provisioning consistent tools, policies and data curation teams. She represents the Bank in several inter-agency data working groups, global data governance bodies and partnership programs. She is an engineer by education and has an advanced degree in Applied Data Sciences.
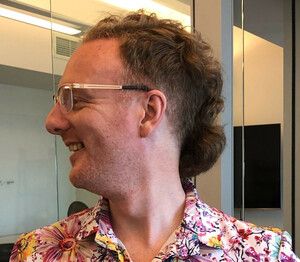
Richie helps individuals and organizations get better at using data and AI. He's been a data scientist since before it was called data science, and has written two books and created many DataCamp courses on the subject. He is a host of the DataFramed podcast, and runs DataCamp's webinar program.
Key Quotes
Not all data is equal. Identify key data elements you need to focus on. What are the crown jewels? Create a priority roadmap about what data you should be monitoring and focus on that.
Everyone uses, and everyone generates data, so we really have to change the way we're thinking about data governance. It must involve all stakeholders. It’s also a shift in mindset, from collecting data to actively using and reusing data.
Key Takeaways
Develop strategies that provide teams with the data they need to innovate and make informed decisions, while also implementing controls to protect data integrity and privacy, ensuring that data is both accessible and trustworthy.
Encourage every member of the organization to take responsibility for the accuracy, privacy, and ethical use of data, reinforcing the notion that data quality is a collective responsibility.
Utilize automated tools and processes to regularly assess and improve the quality of data across systems, ensuring that analytics and decision-making are based on accurate and timely information.
Transcript
Richie Cotton (00:01):
Hello everyone. Welcome to the final panel of today. I am pretty excited about this. I hope you all are too. For everyone in the audience, please do let us know where you're joining from. Let us know if there's anything that you want us to talk about in this next session. I see, oh, saying, no, not the final panel. Sorry. There's a bonus sort of after party session with Joe, our CEO later on after this. But this is going to be good. I enjoy our sessions, but we got four speakers rather than three for you, so it's going to be an extra exciting discussion. So let's see who we got. The chat's scrolling very fast. I'll have to pause this. Okay, we got Anna from Canada, we've got Mark from Philadelphia, we've got Aish from India, we've got Carolina from Toronto, Noah from Florida. I should going too fast.
(01:04):
Too many people here. Andrea from Bogota, we've got Gustavo from Washington and we've got Adam from Romania. All sorts of people there. I'm very, very excited by the global audience. Well, and for everyone joining from around the world, I know some of you in pretty weird time zones for this. So very late, pure, very early. Glad you all made it. Alright, so with that, I think it's time that we got started. So over the last few years, a lot of organizations have been getting really excited about generative ai. They've been building things that think are going to solve all their problems and then they discover that actually the AI is generating garbage. And so when they dig deeper, they... See more
(02:16):
And this is a terrible experience both the data scientist and the audience. Like no one wants to be like, well this is analysis and nonsense. We're wasting our time. So today we're going to learn about how to improve data quality and we generally data governance across an organization. And so for this last panel session, we got four of the finest minds in the data governance space. So first, Stefan Ver Holst is the chief research and development officer and the director of the data program at NYU Governance Lab. He's also a co-founder and principal scientific advisor at the data tank and senior advisor at Marle Foundation. Esther Money is the chief data officer at Safin. She was recognized as the CDO of the year in 2023 at the VE South Africa Awards. And she's also on the list of global data power women in 2023. Amy Grace is the director of military engines digital strategy at Pratt and Whitney.
(03:20):
She spent much of the last few decades running teams working on analytics for predicting the health of aircraft engines. Fascinating stuff and this is something that's got pretty terrible consequences if you get your data wrong. So she's no stranger to worrying about data governance and rounding out our phase and foursome is Malar Raan, a program manager and senior data scientist at the World Bank. She's part of a tasked team that launched the World Bank's Open Data Initiative and was instrumental in creating the World Banks First Data council. So four real experts here. And with that, let's learn about how to improve our data governance. So I think it's worth just defining what we're talking about here. So can you explain what you mean by data quality and what are the business impacts of having better data quality? So Esther, do you want to go first on this?
Esther Munyi (04:16):
Sure. Thank you Richie, and I'm really happy to be here. So what is data quality? Data quality is really about having the right data that you need in the right format and ready for use for purpose at the right time. I use the analogy of data quality is like baking a cake. You need to have the right ingredients, it needs to be in the right amounts, it needs to be available. And I mean if you're baking a cake and the baking powder or the sugar is missing or you don't have the right quantity, then the cake will most likely not come out. So it's really about having data that is accurate, relevant, complete and consistent. And striving for better data quality means that business leaders are able to make better decisions because the reality is if you base a decision on faulty data, you almost likely make the wrong calls which can cost the organization.
(05:20):
And also having better data quality improves the client experience. And by improving the client experience you can increase venues. I work for a bank and one of the things that we put a lot of effort in is improving the quality of our client data. And in order to achieve one of our strategic objectives of being client-centric is we must first understand our clients and we want to understand their behaviors. But to do that we need to study and explore and understand the data that we have around our clients. And the one thing we've also realized is that clients can get very frustrated when we have the wrong information of them. I'm sure most of us are banking with an organization or financial institution and I'm sure you get frustrated if they have the wrong information like the wrong name or they send you a birthday message at the wrong date or they send you communication and you don't receive it because they have the wrong address. So it's important to always have the right information. It comes to clients and I think that other thing is I to have good data quality so that you don't miss opportunities by perhaps not seeing the chances and opportunity to gain more customers and to improve your product offering. And that is really around having a competitive advantage. And so if you don't have the right data and the data's not correct, you're not able to explore those opportunities.
Richie Cotton (07:05):
I really like that analogy of it being like a cake and you mess up the proportions of the ingredients, then yeah, it's going to be a disgusting mess rather than something edible. Alright, so I don't think I've ever heard anyone say, wow, I really love the quality of data at my company. So why does data quality never seem to be a solved problem? Stefan, do you want to go first on this?
Stefaan Verhulst (07:31):
Yes, thanks Richie. And a pleasure to be on this panel, this great panel here focusing on data governance and data quality. And as it relates to your question, I think there are a variety of reasons why data quality is never really an objective that is always or ever fully met. And I think the first one is really about kind of the dynamic nature of data itself. Data is not a static kind of thing. It evolves and especially I would say in the current environment where we have moved to new kinds of instrumentation of collecting data, especially the data that has some kind of a real time quality, there is more opportunity also to really have challenges with some of the data that might not be fully captured or might not be fully qualitative as well. And that also relates to then the dynamic context in which data is being collected, which also means if the context changes, of course the quality might change or the expectations and the requirements.
(08:34):
As Esther was saying, if the cake changes, then the expectations and the requirements for the cake and the ingredients might change as well. And that is especially the case when you start reusing data that was collected for one purpose, for another purpose and then you have different kinds of requirements that also means that the quality requirements might be different as a result never being seen perfect. The other reason is actually that indeed data is not static, but also it's not a thing that is not a result of a process. And so data typically evolves during the data life lifecycle and at every point of the data lifecycle there are opportunities to improve or to decline the quality of the data as well. And I think that's why data governance really and data quality really needs to take an end to end kind of approach when anyway from when you start creating or collecting data to ultimately when you start using the data and the insights that is generated from the data, there is a quality component to every step of the data lifecycle.
(09:44):
And so that also means that given the fact that it's dynamic, given the fact that it's also the result of decisions made across the data lifecycle, means that we not just have to look at data quality from a policy perspective, but really from a cultural perspective. Because I've been advocating on many occasions that data quality is actually the result of a culture of data quality that exists within an organization or within a corporation for that matter. And that really it has to be about a cultural shift towards making sure that data is qualitative, it's not dirty or faulty for that matter and that's really what matters. And then the other shift from my point of view is that we really need to start thinking about data stewardship and how we actually steward data in a way that is aligned with the purpose and that is also then aligned with the requirements that are needed from a quality perspective. So a long-winded answer to your question Richie, but it's of course goes a complex matter and data quality is the result of many decisions, not just one at the point of collection.
Richie Cotton (11:01):
I like the idea that this cake that we're making might want to change over time. You are on different cakes on different occasions, but yeah, it seems like you need that kind of broader idea of data governance and data stewardship if you want data quality. Amy, do you want to add to this, do you have any ideas on how data governance is feeding into into the idea of data quality, staying good over time or getting better over time?
Amy Grace (11:33):
Yeah, I agree with everything Stefan says. In addition, I just think a lot of us are data consumers and we don't always know where the data comes from or who the real producers are of the data that we pick up in different places. And I think we also have a tools first mentality usually express our needs in the way of the data we want to see. So a lot of times we end up with people making local tools to aggregate data and look at it the way they want to, but all of the aggregations and everything are really happening behind the scenes of what they're looking at. And I think a lot of times just the visibility across the enterprise of who has what data and what data is available has been a challenge. So I do think that some of the technologies are helping us to be more aware and concepts like cataloging I think are really important just to make people aware of the data behind the dashboards.
(12:34):
I also think that we're learning to evolve our requests of data to be more in the form of questions we want answered and maybe the generative AI culture is helping us to be as we get experience, A lot of what you get is how you frame the questions. And I think that's been helping us to get better at framing the questions we want to answer to support the decisions we need to make and the actions we need to take. And if then you consider what data do I need to be able to make those decisions? I think we're all evolving in our awareness of the data beyond the dashboard culture.
Richie Cotton (13:16):
Okay. Yeah, I do like the idea that if you're just consuming data, you're looking at a dashboard, you should have an understanding of what the data is underneath the pretty visuals. Excellent. So it seems like maybe we need to have some areas of innovation here. So Mala, can you talk me through what are the main areas organizations need to innovate in terms of data governance?
Malarvizhi Veerappan (13:43):
Thanks, rich and hello to everyone. I really like all this flying hots and Smileys. It's super nice and also the many, many pictures of cakes. It's quite distracting I have to say. But thanks for the question, Richie. I mean I want to kind of take a step back a little bit to just sort of paint the picture of data. Governance happens at different levels at an organizational level, it happens at the national level, at the country, at the highest level, it happens at the international level because data now flows. It's not that data is just used by only a few people or by a few communities or organizations. Data is now everyone uses data, everyone generates data. Everyone uses data actively or passively. So the first thing I think we really have to change the way we are thinking about data governance is that it must involve all stakeholders, whether governments who are using data to improve services or policies, private sector who are creating new innovative products out of the data that they have or opening new markets markets or just individuals in civil societies who can really use the data more effectively to hold governments private sector accountable.
(15:00):
So with this sort of interventions that are going to happen at different levels across multiple stakeholders, maybe I will focus on four or five areas where we think we really need to innovate in data governance. The first I think is really shifting the mindset of collecting, generating data to really use and reuse of data. I don't want to get into this debate on how much data that is being generated. I mean I think we've lost count now and whatever new terms we are using, but there is a lot of data. Granted, there are gaps of course, but there's also lots of data. And the question to ask is whether we are using that data effectively, are we enabling flows of that data across different stakeholders? Are we putting in standards to improve the interoperability of all of this information? So really shifting that mindset towards use and reuse I think is really critical.
(15:52):
And then the second is about to stop. I mean I don't know how many people from the technology team are here, and I'm not saying this in a sort of negative manner, but really looking at data governance is not as a technology initiative because the first thing when somebody says I'm thinking about data is a tool that manifests in their mind. I think now data governance goes beyond creating a technology product. I want to give an example in Kenya where Kenya is doing by the way, many great things, but this is just based off of a study that they did. And this is kind of the situation in many countries where in Kenya particularly this study where they found in 58 hospitals they had across different hospitals, they found 58 different applications that was collecting data on different diseases, on different type of health services that was provided and none of them talk to each other.
(16:43):
So you want to put this scenario in your mind. All of us go to the hospital, I speak about health because I'm currently working in the health sector, so maybe many of my examples are going to be there. But you go to a doctor, they take your vitals that's recorded somewhere, you may have some kind of a accident, you fall, you go to radiology, you get a scan, all of this information is getting recorded. The question to ask is, is that being used actively? Is that being used? Is that information being connected? And for all of that to happen, you can't think of this as a technology issue. The data governance needs to sit outside of a technology initiative where really focusing on new rules of how all of this new data that's emerging can talk to each other, what kind of skills and workforce you need.
(17:29):
Stefan talked about people. I think the people dimension is really key here. Do you have people who are setting standards, new rules of the game? Do you have regulators who are thinking about the broader implications of regulations, of protecting information? Because some of the information we're talking about are really personal data and important to protect. So the point being that is thinking beyond this as being an IT tool and then of course creating a balance of reforms, which is enabling use, but also really important to safeguard information, really protecting, really thinking about cybersecurity, data protection, some of the things that are quite boring and people don't really often talk about those things. And having a really good leadership, which really creates that culture of data use because often leadership teams fail to visualize the tangible benefits from data governance. I think it's important to advocate for that and create that culture of data use and incentives for people to use data more actively.
Richie Cotton (18:36):
A lot to think about that. I think the tricky part is you say nothing is connected, your colleagues need to talk. Talking to people in other teams, that sounds very dangerous to me. Okay, so there's a lot to do. I think we need to get into getting started, but before that I want some motivation. So let's talk about some success stories. I'd like to know if there are any examples of organizations where they're making an effort to improve their data governance and then they've seen some real benefit from it. Esther, do you want to to talk us through some examples?
Esther Munyi (19:09):
Absolutely. So obviously I work for a bank and I mentioned that earlier and one of the things that tends to happen to banks is that we are under stringent regulatory requirements, which demands that we meet certain regulations and legislations. And part of it is ensuring that you have proper governance over your data. But I think the thing that tends to happen is that data governance tends to be seen as this oversight function that's there to come with a stick to come and see that everybody's doing what they need to be doing instead of seeing it as something that's an enabler or a strategic driver for the business. One of the things I can say that for us was a success story was shifting that view and that notion that one, that data is owned by it, it's not owned by it, it's owned by business.
(20:10):
That shift really created the idea of accountability responsibility and also by owning the data from a business perspective, it means that they can leverage it. I love us saying, I forget the person that said it, but when it comes to data management and adopting to data analytics, there needs to be an element of change management. The idea that for business that they own the data that's sitting in a system somewhere is very difficult to fathom and to decipher. But through the process of change management, and back to the quotes that I wanted to say is somebody said change is a threat when done to you, but it's an opportunity when done by you. I forget the person that said that, but it's about taking people through the journey and letting the business users understand why having governance over their data is important. So that's a huge, I think, plus for us.
(21:15):
The second thing is the idea that not all data is equal. There's this idea that you need to go and govern all the data, and that's not necessarily true because some data that you might have or data elements that you might have in your organization is actually not useful or fit for purpose. It could just be unusable really. So it's about identifying what are those key data elements that you need to focus on? What are your crown jewels and then focus on that. So one of the things we've done with data quality is create that roadmap around what data should we be overseeing, what data should we be managing and what data should we be monitoring and maintaining from a data quality perspective. The other thing around when we talk about not all data is equal is also true to data quality. If you take the example of the cake, you might have a scenario where you put in a little bit of sugar, not enough, but it's still edible.
(22:22):
But if you do not put any baking powder or you do not put any flour into the cake, it's not useful. So what we've done is we've also realized that there's a level of tolerance around data quality. And that's what we've applied in our data quality framework where we've tried to understand based on the different data quality rules and data quality metrics, what is the tolerance for the business? Because that way when business is making decisions based on a certain tolerance levels, they know that when they make that decision, it's based on a certain standard which they've defined. The other thing that I think that has been very successful is realizing that the human element around governance is often overlooked. We tended to stick to the technology to the data itself and not really looking at the people aspect. So we've really also started to shift that and frame the way we look at data governance, but focusing on the people and that means ensuring that the data affluency or data literacy of key people is elevated in order to improve our data quality and to ensure that data governance is embedded in a way that's useful
Malarvizhi Veerappan (23:44):
For our business.
Richie Cotton (23:48):
A lot to think about there. I like the idea that you need to decide which things are the most important, which data sets the most important, and what your tolerance for quality is for those. Because there's a lot to go on. I'm trying to book out what the first step, Amy, do you want to talk through when you're right at the start? How do you begin improving your data quality?
Amy Grace (24:12):
I think some of the most important first steps is to have a burning platform. There has to be a need for change. People have to say, this can't go on because their experience with the data is just not working. Another thing in a company that's invaluable is to have strong executive championship. There's no support to having a courageous leader at the top who will empower people who want to change. I think another thing that's important is to have a data governance professional. So somebody who can help teach us the ins and outs of data governance. I also think it's equally important to have case studies that'll help to teach the people in the workforce, especially the executives that are going to have to drive some of these things down through the organization case studies that'll teach them why we should care about data governance and what the consequences and how it's holding us back.
(25:18):
And then lastly, when we started our data governance council, I think somebody else mentioned the importance of change management. We actually have a change management specialist working side by side with our data governance lead. And the most important part of this are engaged, committed forward thinking business partners because like Esther says, they own the data or they are most intimately familiar with the data we're asking them to take on new roles and to have those people come and be committed as opposed to just compliant is the key I think to really take off and start our journey.
Richie Cotton (26:10):
I like that you mentioned there should be some sort of executive leadership involved in this. Maybe we should talk a bit more about which teams and which roles need to be involved in any sort of data governance program. Do you want to take this?
Malarvizhi Veerappan (26:29):
Sure. Na. So I often say that we want to think about it more in terms of the functions because each organization creates its own team or I guess the role remains the same, but it's often difficult to create new teams, depends on the fiscal constraints of the organization, again, at different levels at the national level or at an organizational level. But importantly, I think Amy touched upon some of those roles already in terms of, and I think Esther also in the sense that having first the data governance, having that sort of leadership from the top is important. You kind of need both. You don't want it to be a very compliance oriented sort of tone that you set for data governance. So you have a leadership that really shows that this is beneficial for everyone and you're kind of recruiting everybody to this agenda. And so you need that executive committee that is owning this so it's sustainable in the longer run.
(27:26):
Then you have to have different also dedicated roles for people who are going to be framing standards around for data governance. You need business domain experts who understands the data, who actually, so it's not data for data's sake, but really how at the end of the day, how are you going to use that data to improve any type of business outcome. It could be from the government side, improving policies or reducing poverty or providing better services. From a private sector perspective, it could be improving their own business outcomes. So even from an individual's point of view, if you had access to your own health record, you can take better decisions, for example, on your health or on your financial outcomes. So it's about really having those business domain experts as part of the committee. I mean I think Stefan already talked about this new sets of roles that are getting created in organizations called data Stewards, whose role is really to look at data and see how that data can be used in the organization, how data can flow across different departments.
(28:37):
Often you have siloed use of the data data from say a finance department is not really being used, it could be used for some other purpose that would be the responsibility of the data steward. And another group of people is the legal team in an organization or regulators or data protection officials at the national level who are deciding on these regulations and policies that is really standardized. I know we all love lawyers, but as much as we love them, I think it's really important to still engage them and sort of really bring them along as well because they have, like Esther said, sometimes you really have strong compliance requirements, but I think somewhere you have to see the balance to see how you can bring them along to be able to use this data efficiently. And then of course, people who are very looking at measurements and very technical issues like anonymization of data, some of these areas are still being explored now that we are bringing in very many different types of information like geospatial cell phone records. So having a team that's technically aware of how you bring some of these anonymization techniques or data integration techniques and continuously thinking about that in a systematic manner is also important.
Richie Cotton (29:57):
This is interesting because I was kind of expecting the answer, okay, we've got executives, we've got the technical data people, we've got business people, but it's actually, it goes beyond that. You need legal people as well, and then even people outside your organization like governments creating regulations. So it's a very much a team effort there. Alright, so I feel like a lot of the ideas around data governance are going to be the same from one organization to the next and you shouldn't be having to reinvent the wheel from scratch. So are there any principles or frameworks around data governance that you can leverage? Stefan, do you want to take this?
Stefaan Verhulst (30:37):
Yeah, sure. And again, this is a wonderful panel and also by the way, a wonderful chat, meaning there's a great set of lessons even learned from just looking at the chat. And so I'm not sure how much I have to add here, but one of the frameworks that we have developed in order to really demystifying data governance is something what I call the five Ps of data governance, which really is about purposes, principles, processes, practices and positions. And I think we have discussed a few of them already because to a large extent, from my point of view, data governance is actually a set of practices, positions and processes to meet a purpose that is aligned with a set of principles. And I think if you think in terms of those kinds of five Ps, then you basically have kind of all the ingredients for the cake that Esther has been baking here.
(31:41):
And it also means that we really have to be crystal clear. And it goes back to your question rich on where would you start? And I always anyway recommend organizations or anyone who wants to develop a data governance structure to really start with the purpose because that's really where it all comes down to. Because otherwise why do you need governance if you have no purpose that you seek to establish on meat? And so a crystal clear purpose, but then in order to achieve that purpose, you will have to make decisions. And so then it's going to be very important to have a set of principles that will align those decisions in a way that meets the purpose, but that it's also principle based. And so here, of course, I'm not going to go into the full fledged set of principles that you can apply. And there are of course well established such as the fair information practice principles, which anyway were developed 30 years ago, but are still anyway, some of them are still pretty sound and actually should be retained.
(32:52):
But you also have a set of new principles from my point of view that have entered the space. One of them is actually equity and inclusion, which I think needs to be more included in data governance, meaning that, anyway, how do we make sure that the data benefits everyone to a large extent in a way that is also inclusive, but also the principle that we have worked on, which is kind of digital self-determination, which is of course specifically more relevant for personal data, where at the same time you not just rely on content, but you also rely on a kind of additional areas of agency where individuals can also actually provide their preferences and expectations on how the data is being used to serve them and to serve society as a whole. And so these are a set of principles that can be used to then inform the processes that need to be in place to make decisions.
(33:53):
And I think Malara was referring to all the kind of ingredients and the positions that need to be in place, but you also need to have decision processes because by the end of the day, you need to make decisions on how you actually go about the purpose that is aligned with the principles. And here I think it's super important to also make sure that those processes are seen as legitimate and at the same time effective. And I think that's another kind of element of the framework as well. So in some Richie, I think there are five Ps that one may want to address the purposes, the principles in order to make decisions via processes that ultimately then need to be implemented through practices and then dedicated positions that can oversee whether those practices align with the decisions and the principles as well.
Richie Cotton (34:53):
Oh man, purposes, principles, something, practice processes. I think I've got four out of five.
Stefaan Verhulst (34:59):
I gave you a monic to make it easy.
Richie Cotton (35:01):
Okay. Alright, everyone has to watch the recording back and repeat that phrase over and over until they got all five. Thank you very much. We're out of time for my questions already. This has gone by so fast. Alright, we've got some great questions from the audience though, so let's dive over to those now. The first question comes from our niche saying how do you balance the necessary data governance with agility and accessibility? Can we avoid creating processes that stifle innovation, make data difficult to use? Alright, so yeah, how do you keep yourself nimble and agile while having good data governance? Who wants to go first on this? No takers. This is a possible question to answer.
Malarvizhi Veerappan (35:54):
I can maybe take a stab. Okay, cool. I think it's a very, very pertinent question. I think that's a struggle for everyone to kind of balance what we say, how do you balance enablers, which is about enabling use while safeguarding and protecting information, but also you're looking at it more from let's not make it too compliance oriented, that it's just so hard to innovate based on that. I think it's a process in the sense that, I just want to go back to what Esther said. Maybe I'll just connect Esther's point with what we did at the World Bank as well. We wanted to show, I think you can do that by illustrating value. For example, at the World Bank about five or six years ago before we really ramped up our data governance initiative, a simple question would be as staff, if I joined the organization, what data I had access to.
(36:52):
The way we would do that is someone would find data is they call someone and call someone. There's a phone tag that you play, you don't know where to go, you don't know what data you had access to, you didn't know how you can access it. You don't know what are the terms under which you can actually access that data. People were afraid to share information. There's this famous phrase by hands rosling that everybody must have heard called a database hugging disorder. I think that was a serious disease for us at the bank, but I think we've,
Richie Cotton (37:19):
Sorry, I haven't heard this phrase. Database hugging disorder.
Malarvizhi Veerappan (37:22):
Hugging disorder. Yes. It's when you decide to hug your database and you don't want to release it or share it. So it's hands, I didn't coin the name. But again, going back to the point is that I think you want to show value. I mean there are some things that organizations do like the World Bank did as well as the first step is we try to understand what data we had and that manifests itself in different ways. Sometimes it's in the form of a data catalog where you then understand what are your high value data sets, and then you really focus on governing those and you're able to show what kind of innovation and value that you could bring in some other cases. I will give you another example where these are real deliberations. You might get frustrated about why you're not able to use certain data, but it's just because of the nature of regulatory environment we are in or we just don't have standards.
(38:18):
So we just have to proactively start setting them up. In the case of Covid for example, I think everybody saw that how we really struggled to use information, even high income countries that had very strong data systems really struggled to use information from their health systems or from mobile phone operators because we didn't have a regulatory environment to access that information or we didn't have technical standards that talk to each other. So by focusing on them I think, and bringing in change management is something then we hope to reach that balance between that enablers and while protecting and having stringent processes that protect information.
Richie Cotton (38:59):
Absolutely. So that covid example where you've got a ton of data, but actually making use of that data is very difficult. That seems like a problem everywhere. It's like, okay, yeah, you're not actually having an impact unless you can make use of your data. Okay, so next question comes from Lawrence. So Lawrence asks, how do you actually measure the quality of data? How do you know if your organization's data is good or bad? So yeah, what's the scoring system here?
Esther Munyi (39:32):
I can take that. Sure. Lawrence, I think what I can say is the approach that we've taken is we look at data quality based on three categor. One is industry standards. So for example, when you have quality, you want to measure your data. For example, currency data. Obviously there's industry standards around how currencies should look like, how they're named. There's the ISO standards. So we try and get industry standard type of rules into the way we measure our data. The second category is regulatory. So I think also I saw in the chat somebody talked about gp, GDPR in South Africa where I am, and it's basically taking those standards from a regulatory perspective and applying that to our data quality rules. Then the third one, which is the most important one, is the business context. And the business rules is your data meeting the rules of your business processes.
(40:42):
And then some of the ways we measure that is really, and the approach can vary. We've adopted the D Dharma D approach, we've taken the different data quality dimensions and we've tried to create rules around those different dimensions. And then the other thing that we had to do is make sure that we workshopped with our business users and we also engaged with different leaders from different areas of the business, like Mala mentioned, legal compliance risk teams to give us also a different perspective and lens to the data. Because also what tends to happen is that when you look at it only from a business context and you ignore the other factors, for example, what is needed for financial management and reporting what's needed from a risk management perspective, you miss out on some of those pertinent rules that you should be measuring. And obviously for us it's also visualizing those metrics and making sure that it's accessible to people. And one thing that really worked for us is even when we build reports, we add on a layer of having a data quality metric for that report. So you measuring the data that's been used in that report so that the decision maker knows the level of quality of the data that's in that report in order to make decisions. So there's different approaches. We've just adopted the Dharma D approach.
Richie Cotton (42:18):
Oh man, I do like the idea of showing data quality in the dashboard. So the person who's down the street, you can see that that's kind of terrifying though. If you get a low score, it's there going to be some funny questions asked, why is this dashboard existing if it's not very good quality. Alright, we are basically at time, so before we finish 10 seconds each on, how do you do data governance better? So final advice, Amy, would you like to go first?
Amy Grace (42:51):
I would just say start small, deliver value, drive awareness, and I liked the thing I saw in chat. Use the business as your word of mouth ambassadors and it'll spread. I like that one a lot.
Richie Cotton (43:08):
Excellent. Yeah, that's very cool. Mala, what's your final advice?
Malarvizhi Veerappan (43:15):
I mean, I really want to second what Amy said in terms of start more and then you really become agile and improve. I do want to add three principles that we talk about when we talk about data governance, which is value getting value out of data, look at it through a value lens of how you can unlock that value by reuse and use. And the second is equity. A lot of data that we use today is really used only for very to the benefit of certain groups of people. So in how you deliver services using the data, I think it's really important to have the equity dimension that everyone benefits from the use of the data that there is. And the last is building trust with all of the exposure to data that I'm sure you're all reading in the news about data security issues, data breaches. I think it's really important to safeguard data which will build further build trust in the data that we are producing, along with being very transparent about how we are processing data and how we are using data and around the data quality dimensions. Good luck to everyone.
Richie Cotton (44:24):
Good luck. You'll need it. Alright Stefan, what's your final advice? Yeah,
Stefaan Verhulst (44:31):
Well meaning it's always hard to narrow it down, but I would just pick up on something that Amy actually mentioned earlier as well is that it's super important to formulate the purpose well, which also includes formulating the questions well for which you then need data. And then you also know to what extent does it need to be governed? To what extent can it be made equitable? To what extent can it actually be done in a trusted way. I would, as some of you might know, I've been advocating for actually question science to compliment data science because we really need to do better in how we go about formulating questions because that's where it all starts.
Richie Cotton (45:10):
Alright, wonderful. Yeah, get better at asking and answering questions. Esther, final piece of advice from you.
Esther Munyi (45:22):
I think everybody said great points. I think for me is also just start where you are, assess your current state and maturity. I think it's important to know where you are, what the gaps are, where your shortcomings are, what strengths you have already that's in your organization. It doesn't make sense starting a journey where you don't even know where you are in that journey. So it's important to understand where you are and what your readiness is, what your organizational readiness is, and what risk tolerance you have in the organization.
Richie Cotton (45:54):
Oh yes, understanding your data maturity. Very important. Alright, we are well over time now and everyone needs to jump the final session, so I would have to wrap up quickly. Just thank you to all four of our speakers. That was just magnificent stuff. Really, really informative. Yeah. Thank you all.
Esther Munyi (46:11):
Thank you for having us.
Richie Cotton (46:15):
Alright. And for everyone in the audience, please do jump to the final session. It's going to be a good one. All right. Right.